Sports Betting and Data Analytics: How Big Data is Revolutionizing the Industry
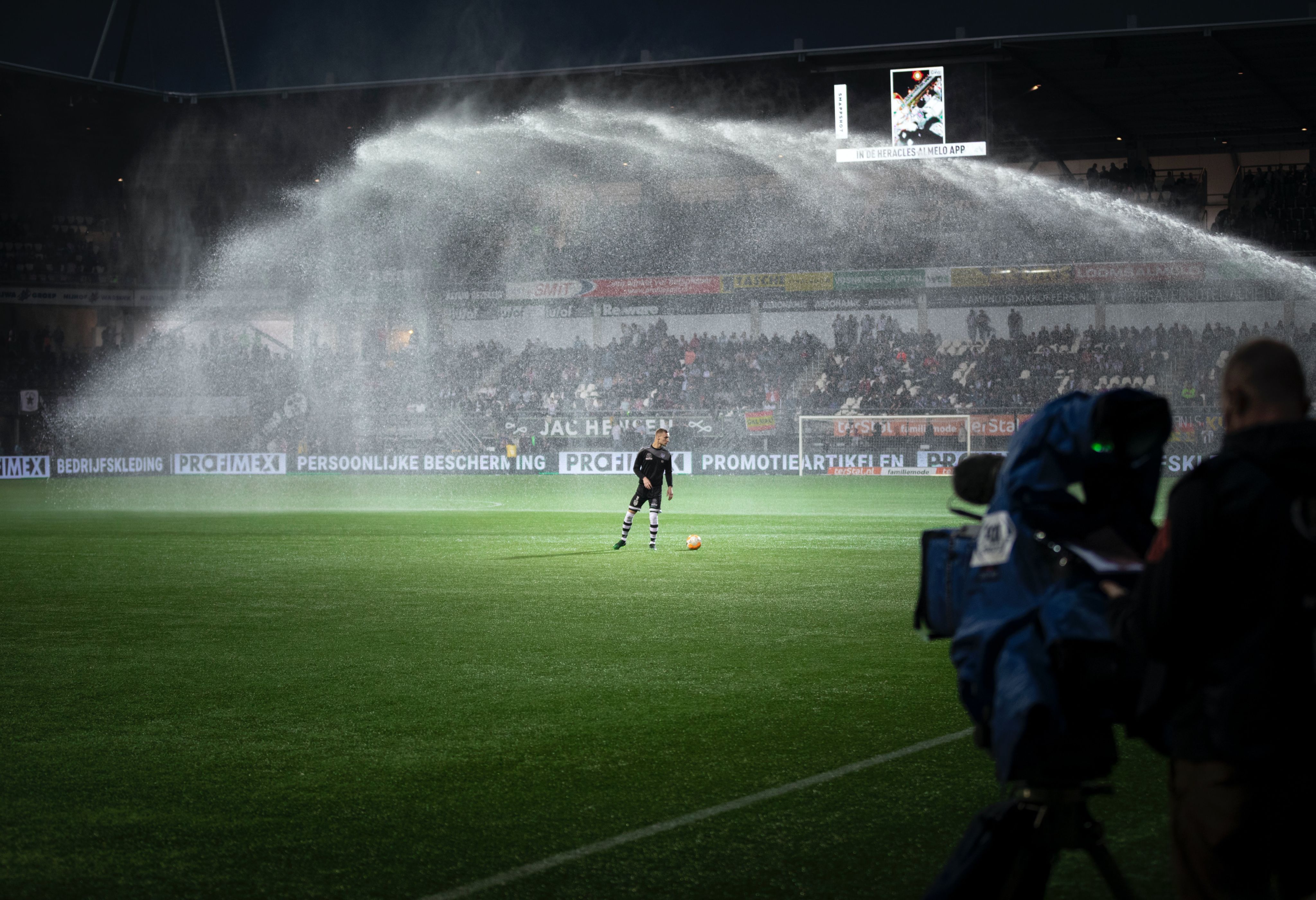
We organize events not only on topics that we specialize in. In February, we gathered specialists in the use of machine learning in sports. It's amazing how many processes connect these two fields - data analysis and sports - and how many unsolved problems arise at the intersection between them. Here is a presentation by Dmitry Dagaev, Deputy Rector of the National Research University Higher School of Economics.
The Rise of Data-Driven Sports Betting Strategies
Today, I will briefly talk about the tasks that are already being solved using data analysis in sports. We will see that the interaction between agents is the key factor that allows us to solve these tasks.
In general, data circulation in the sports industry is organized as follows. Firstly, there are sports federations and leagues that determine the rules of the game, under which sports competitions and sports economies operate.
The problem is that these leagues, competitions, and the economy are very complex structures. And when making optimal decisions, of course, we want all of them to be optimal, but federations face the need for precise analysis. This is not always possible. Often, to get an accurate answer that maximizes a certain value, one needs to conduct a great computer simulation or some regression analysis. Of course, the federation cannot always do this on their own.
The rules are defined, and clubs or individual athletes are forced to live by these rules. The next arrow also characterizes a certain range of problems. We played a match or a chess game. In theory, we can extract a huge amount of data from a match. Until recently, data extraction encountered serious problems. How to do it? The development of modern computer technologies has allowed, for example, in strategic games like chess or go, to defeat the strongest athletes. And in games like simpler versions of checkers or poker, to find practically exact solutions.
Accessing Reliable and Comprehensive Data Sources
Data extraction in tasks that require image analysis is a quite expensive affair. Therefore, in the market, companies have emerged in the last 15-20 years that deal with filming football or hockey matches and then successfully sell this data to clubs, federations, or researchers.
Another problem is analyzing the data. This is where the academic community comes to help. Research departments often exist in sports clubs themselves, or in companies that deal with data extraction, or in universities as separate research centers. And they provide recommendations based on the analysis of the collected data. This is how this system is generally structured.
Why analyze data?
There are two main goals. For teams and athletes, it allows increasing the probability of winning in an individual match. On this slide, we have Jens Lehmann. Perhaps the most famous example is when in 2006, the crib sheet he pulled out of his socks allowed him to save two penalties from the Argentine national team and advance to the next round of the World Cup.
The second generalized task is to improve financial performance. Questions related to increasing the sale of broadcasting rights for matches. This requires accurate analysis of consumer preferences, pricing policies. Here, data allows finding optimal answers.
Overall, clubs may be interested in analyzing their opponent's strategy to find the optimal response.
In the early 2000s, the famous article "Professionals Play Minimax" by Ignacio Palacios-Huerta appeared, which showed that in reality, players taking penalty kicks behave very similar to what the theory predicts, and this allows clubs, by analyzing the data, to find optimal responses to the expected strategy of a particular player. Including when he deviates from the equilibrium strategy and tries to use something different, it allows successful play against it.
Transfers, finding underestimated athletes, is also a very important task where breakthroughs are now being made. Each club can formulate its own specific request for the type of player they need. It is understandable that there is a large number of athletes on the market, each player has a certain transfer value, and for each player, a large volume of statistical data can be collected regarding their recent performance. However, it's not enough to just look at generalized statistics; it is necessary to match this data with the specific needs of the club. Therefore, each club has to search for those players who are optimal for their requirements.
Assessing the effectiveness of an athlete, how to structure their contract, how to find the optimal part of bonuses that need to be paid, how to ensure that they exert maximum effort in each specific match—these are all important tasks that clubs are currently interested in.
The Importance of Effective Bankroll Management
From the perspective of federations or leagues, the tasks are slightly different. An important task is the design of competitions, how to make the league as competitive as possible. Because the higher the competition, the more attractive the league becomes to viewers.
How to regulate the league, how to establish optimal rules? What will be the impact of limits on foreign players? We don't just want to claim that it will allow our national team to perform successfully; we want to try to model the situation and provide a more or less accurate answer based on predictions, simulations of this complex system, which in its complexity can be compared to the economy of a country.
Another task that has been actively addressed recently is the detection of match-fixing. The illegal betting market is growing rapidly. If the betting market as a whole is already estimated in the billions of dollars, the illegal betting market, even if it occupies a fraction of that, still represents a significant volume Exm.nr.
The fight against doping is especially relevant today. How can we make predictions about a sportsman's use of doping, even if we cannot directly detect a particular chemical element? Data analysis also helps in this regard.
In recent years, a large number of investors have entered this market. The cost of solutions is increasing. So, there is a lot of potential in this field.
Sports betting is an activity that has captivated enthusiasts around the world for centuries. The thrill of predicting the outcome of a sporting event and potentially earning a profit has made it a popular pastime. While sports betting is often associated with luck and gut feelings, there is a growing trend towards using data analysis to gain an edge in this competitive arena.
Data-driven sports betting strategies leverage the power of statistical analysis and historical data to make informed predictions about the outcomes of sporting events. By analyzing factors such as team performance, player statistics, injuries, weather conditions, and other relevant variables, bettors can make more accurate assessments and increase their chances of success.
One of the key advantages of data-driven sports betting strategies is their ability to identify patterns and trends that may not be apparent to the average bettor. By analyzing historical data, researchers can uncover insights that can inform betting decisions. For example, they may discover that a certain team tends to perform exceptionally well in certain weather conditions, or that a particular player consistently performs better against certain opponents.
To implement a data-driven sports betting strategy, it is essential to have access to reliable and comprehensive data sources. Fortunately, in the digital age, there is a wealth of information available at our fingertips. Sports databases, statistics websites, and advanced analytics platforms provide valuable data on teams, players, and various performance metrics.
Analyzing this data requires a combination of technical expertise and domain knowledge. Data analysts and sports enthusiasts work together to develop sophisticated models and algorithms that can process and interpret vast amounts of data. These models can identify patterns, calculate probabilities, and generate predictions that inform betting strategies.
The Future of Data-Driven Sports Betting
One popular approach in data-driven sports betting is machine learning. Machine learning algorithms can analyze large datasets, learn from patterns, and make predictions based on historical data. This technique has been successfully applied to various sports, including football, basketball, tennis, and horse racing. By training machine learning models on historical data, bettors can gain valuable insights and make more informed decisions.
It is important to note that data-driven sports betting strategies are not foolproof. While they can improve the odds and increase the chances of success, there is always an element of uncertainty in sports. Unexpected events, injuries, or changes in team dynamics can influence the outcome of a game. Therefore, it is crucial to combine data analysis with careful consideration of other factors, such as team news, expert opinions, and intuition.
In addition to data analysis, effective bankroll management is essential for long-term success in sports betting. Setting a budget, managing betting amounts, and avoiding reckless bets are key principles to follow. It is important to treat sports betting as a form of investment rather than a gamble and approach it with a disciplined mindset.
As the field of data analysis continues to evolve, we can expect even more sophisticated strategies to emerge. Advancements in technology, such as the Internet of Things (IoT) and wearable devices, offer new opportunities to collect real-time data and gain deeper insights into player performance and game dynamics. This data-driven revolution in sports betting has the potential to revolutionize the industry and create exciting new possibilities for both bettors and sports enthusiasts alike.
To summarize, the field of data analysis in sports offers numerous opportunities and challenges. By leveraging data, sports organizations can improve their decision-making processes, enhance performance, increase revenue, and provide more engaging experiences for fans. The collaboration between data analysts, clubs, federations, and researchers is crucial in unlocking the full potential of data-driven strategies in sports.